Artificial Intelligence Will Write Code at Lunch
Solving AI's Power Crisis
With AI technology development not slowing down, there's only one solution: for power-hungry tech to consume when renewables are at a surplus, and energy is going to waste.
AI-assisted software programming is energy-intensive and expensive. So dynamic pricing models that make it cheaper to operate when power is abundant, at scale, could be worth billions.
This timing shift aligns perfectly with solar energy production peaks: AI will write code at lunch.
Contents
- A power crisis is coming
- Power prices give us the signals to avoid disaster
- Why doesn't demand already listen to power price signals?
- AI power demand will be intelligent
- The strong market economics of power-aware GPU clusters
- Software programming is a key high-intensity AI use case
- How to practically make GPUs aware of power costs
- AI meets our climate goals
- Summary: Power-aware pricing is the future for powering AI
A power crisis is coming
As global use of AI technology continues to trend up, so too does demand on electricity infrastructure. A severe power crisis looms on the horizon, clearly visible in the base load deficit shown in the chart below. Global electricity infrastructure simply cannot meet projected AI demand while simultaneously managing the renewable energy transition.
We've reached a point of a fundamental mismatch: AI is adding consistent base-load demand to the grid, precisely as we're removing traditional base-load supply, such as coal. Renewables remain too intermittent to reliably fill the gap, and battery storage at grid scale is prohibitively expensiveiStorage has an enormous role to play in the energy transition, but the economics of storage depend on cycle times. Storage that supports energy shortages that occur once a month or once a year will only cycle once a month or once a year. As such the assets supporting those tail risk energy shortages will make significant losses. It is possible we move to capacity markets and spread the cost across the generation fleet, however it is still a significant burden. . Yet fossil fuels face regulatory extinction, and nuclear development is moving too slowly to meet immediate needs.We can't transform supply, so the only viable solution is to fundamentally transform demand — making AI tools responsive to power availability. If we are to realize the transformative potential of AI, the industry must think carefully about its relationship with energy.
The challenge is urgent
This is not a hypothetical problem. In Singapore and Ireland, energy grids have reached their limits, forcing a moratorium on new data centers. Elsewhere, we've seen data centers resorting to backup diesel generators at times of grid stressiNiangjun Chen, Xiaoqi Ren, Shaolei Ren, and Adam Wierman. 2015. Greening Multi-Tenant Data Center Demand Response. SIGMETRICS Perform. Eval. Rev. 43, 2 (September 2015), 36–38. https://doi.org/10.1145/2825236.2825252 https://niangjunchen.github.io/papers/MAMA_colodr.pdf. Needless to say, that's an unsustainable practice they rarely publicize.
Leading technology companies also recognize the challenge, with major AI players backing grid-stabilizing initiatives like DCFlexiDCFlex is backed by Google, Nvidia, Meta among others. https://msites.epri.com/dcflex, and Google pioneering demand-response technologiesiSupporting power grids with demand response at Google data centers. https://cloud.google.com/blog/products/infrastructure/using-demand-response-to-reduce-data-center-power-consumption. Meanwhile, startups (like ours) are emerging specifically to address this challenge. As more AI deployments roll out, and more electricity grids reach capacity limits; and as media coverage intensifies and public awareness grows, the urgency of the power problem will only increase.
Former Google CEO Eric Schmidt recently warned Congress that AI demand could account for up to 9% of global grid power consumption and that action is needed now to support that demand. Schmidt believes strategic use of AI is of strategic national importance, and could change the balance of power globally between countries, so the stakes are high to get this rightiFuture of AI Technology, Human Discovery, and American Global Competitiveness
https://www.youtube.com/live/HXoXMETZUiE?t=1760s.
AI economics are driven by energy
AI's economics are already driven by energy, and will become even more so. Data center rack density is growing exponentially, from traditional 6kW racks to next-generation systems approaching 600kWiNvidia's Jensen Huang, Ian Buck, and Charlie Boyle on the future of data center rack density https://www.datacenterdynamics.com/en/analysis/nvidia-gtc-jensen-huang-data-center-rack-density/. This shifts the cost equation even more towards power. Electricity costs will increase, but rent will stay relatively fixed.
...Eventually the cost of intelligence, the cost of AI will converge to the cost of energy and it'll be how much (energy) you can have. The abundance of it will be limited by the abundance of energy.— Sam Altman, Senate Hearing on AI Competitiveness
Simultaneously, as Nvidia CEO Jensen Huang notes, GPU depreciation is accelerating with each product cyclei"..when Blackwell starts shipping in volume, you couldn't give Hoppers away" — Jensen Huang, Nvidiahttps://www.datacenterdynamics.com/en/opinions/are-we-approaching-the-sell-by-date-of-ai-clouds/, further increasing power's proportion of total operating costs. Today's AI fleet is made up of modern, newly-released GPUs. As the market matures, the fleet will be dominated by much cheaper, heavily depreciated silicon. Energy will become the only cost that matters. And then, one principle will define the industry: The ability to deploy AI will be directly linked to the availability of power.
Power prices give us the signals to avoid disaster
Many countries already have advanced power markets with 'peak' and 'off-peak' pricing, which provide strong incentives to respond to grid availability. When power is scarce, more expensive generation such as gas turbines must deliver, which pushes prices up. When power is plentiful, generally because we have renewable excess, prices drop (potentially even becoming negative, meaning customers are paid to consume power). At lunchtime, in solar-heavy markets, power essentially becomes free.
The AI industry must dance with the duck
The 'duck curve' illustrates these dynamics. There are two main points where power is scarce and prices rise — the tail and the top of the head (or under the chin, depending on how you look at it). Outside of these times, renewables serve us well.
In many markets, we have an excess of renewable energy during the middle of the day that isn't being utilized. As solar and wind technology has soared ahead, we've built vast capacity for renewable energy without having systems sophisticated enough to utilize the intermittent output. This asymmetry creates the 'duck curve paradox': Clean energy supply peaks during the day, while demand is at its lowest, meaning it goes to waste, while fossil fuel plants still fire up at dawn and dusk.
Power-hungry AI servers don't care what time of day they're online and working their hardest. If we can tailor energy demand to respond to these power pricing signals, we can reduce grid stress in peak times, open up room to add more renewables, and save on the financial and environmental costs of high-energy AI activities. To avoid the looming power crisis, there is no other option than to innovate in line with power supply — to dance with the duck.
Sourcing energy is often viewed as a 'trilemma'; a balancing act between sustainability, reliability and affordability. A smarter approach, matching demand to supply, solves for all three elements at once. Smarter demand means AI servers will soak up excess renewable energy, demonstrate demand, and pave the way for more deployments and more facilities, thereby accelerating the green energy transition.
Deregulated energy markets are the missing link
To realize the potential of this, we need deregulated energy markets. Unlike rigid centralized systems, these markets will generate precise price signals that reward flexible consumption. Regions clinging to outdated or over-regulated market structures will face an impossible choice: reform to allow for price-responsive AI workloads; or watch their tech economies suffocate under power constraints. The coming AI boom will validate deregulation not as ideology, but as a computational necessity.
Why doesn't demand already listen to power price signals?
So, why don't we already operate in this way, tailoring power demand to supply? The problem is in the delivery. Energy retailers, the businesses selling power to customers, don't pass pricing signals on to consumers. Rather, they hedge volatile power prices into simple flat rates.
Flat pricing is arguably simpler for customers, and it made sense in a primarily coal-powered world, when demand and supply were relatively flat, too. But flat prices today offer no incentive for smart power consumption, and no penalty for consumption during periods of grid stress. The energy transition has made things complicated, and supply more intermittent. The way we generate power has evolved. The retail model has not.
Data centers must be smarter
At the same time, customers can be apathetic. Households are slow to change their behaviors, and the promise of a dollar saved here and there may not be enough of an incentive. If we're looking for true demand elasticity, where price swings can drive large behavioral changes, we find it not in the mass market's dimmable lights, but in data centers. A single hyperscale facility can have daily power needs equal to those of a small town. Here, even a 5% differential in energy pricing can be worth millions.
Data centers run on systems and systems can listen to signals. Currently, however, the computing industry prioritizes reliability over energy optimization, so nobody has focused on developing this capability, or placed much value on the benefits. But many computing workloads can be easily shifted, or staggered. For example, cloud storage servers have built-in redundancy across availability zones. Many batch or backup-related tasks are not time-dependent, and latency is not as important, meaning these tasks could be done from different regions, depending on power availability.
We're seeing apathy in action, and there haven't been great incentives to do anything differently. It's only as the AI boom drives energy usage, and power becomes both a larger part of the cost base, and more scarce, that this is becoming pressing.
As the energy crisis intensifies, the technology industry will have to proactively develop smart consumption solutions, and the computational sector will have to evolve towards power-aware operations. The only question is whether this transformation will be strategic or reactive. Once some computing use cases start to become power-aware, it won't be long before the grid operators, the media, and society as a whole will expect all of computing to evolve.
AI power demand will be intelligent
AI is different from typical computing because of the extreme power required by the chips. AI economics are predominantly power driven as a result. AI looks a lot more like crypto than cloud, and crypto mines represent the most sophisticated power-aware computing operations on the planet, often directly using spot-passthrough pricing.
Not all aspects of AI will be power-sensitive. User-friendly subscription models, for example, are designed for low-intensity AI use cases. AI training is another example of such an arduous and fault-ridden task that power optimization hasn't been a priority. If AI is to change economies and reinvent this world, we need high-intensity AI deployments that will be very power-sensitive.
When is AI high-intensity?
We define high-intensity as many AI iterations per task. For example, any agent-driven use cases would classify as high intensity. High interaction use cases such as voice will also classify as high intensity. Test-time compute is also seeing the intensity increase even for basic tasks.
High intensity use cases will be using upwards of $10 per day worth of LLM capacity, which is the kind of usage we should expect if jobs are automated in society. Because of their scale, high intensity use cases will dominate AI revenue, and the economics of the AI industry. For high-intensity use cases, optimization of cost, latency, and computing costs then become very important.
How the high-intensity LLM model works
Early signs suggest these high-intensity workloads will use LLM routers such as OpenRouter or Requesty to manage the cost base. A router is a business that takes a single request and forwards it to an LLM provider, based on different criteria. While the customer, or requester, pays a flat rate, the router saves money by selecting the appropriate LLM for the task — a router won't use an advanced LLM for a basic task, for example. Routers also ensure reliability, because there are fail-safes integrated, and they make it easy for businesses to manage the ever-evolving array of LLMs an application might use.
The routing operating model also makes it very easy for an LLM provider to adjust their prices based on different power costs; they can simply bid a different price to the router when their cost base changes.
If a GPU's power becomes too expensive, it will lose the volume, and more efficient GPU clusters will gain. Once high-intensity workloads become dominant, most AI computing capacity will come from routers. In just a few years, GPU clusters will be earning most of their revenue from these routers.
We need a new GPU ecosystem
Application layer companies like Meta have a strong incentive to keep competition for LLM's healthy. LLM's form part of their cost base, and by funding open source models they create price competition to keep these costs downiFor example, if Meta projected AI costs to be $20 billion of their cost base (out of $120 billion total expenses) in the future, and a cutting edge model costs $1 billion to train, and giving away the open source model brings the cost of AI down to $10 billion per annum, it is a very worthwhile investment on Meta's part.
"We expect full year 2025 total expenses to be in the range of $114-119 billion. We expect the single largest driver of expense growth in 2025 to be infrastructure costs, driven by higher operating expenses and depreciation(1). We expect employee compensation to be the second-largest factor as we add technical talent in the priority areas of infrastructure, monetization, Reality Labs, generative artificial intelligence (AI), as well as regulation and compliance. We anticipate our full year 2025 capital expenditures will be in the range of $60-65 billion. We expect capital expenditures growth in 2025 will be driven by increased investment to support both our generative AI efforts and core business. The majority of our capital expenditures in 2025 will continue to be directed to our core business."
https://investor.atmeta.com/investor-news/press-release-details/2025/Meta-Reports-Fourth-Quarter-and-Full-Year-2024-Results/default.aspx. As such, the GPU ecosystem and routers will have a large array of models and complexity to manage, with a strong need for price optimization. Some (like OpenAI's Sam Altman) argue closed-source models will maintain superiority through cutting-edge performance. However, given that more of the economic value will be captured by the application layer, and their incentives are to fund open source, it is likely that open source is a significant component of LLM capacity going forward.
The GPU market is evolving into a complex ecosystem with varying tiers of providers, distinguished by their technical capabilities, sophistication, security standards, and service quality – as well as appropriate scale for customer needs and locationiThe GPU Cloud ClusterMAX™ Rating System | How to Rent GPUs https://semianalysis.com/2025/03/26/the-gpu-cloud-clustermax-rating-system-how-to-rent-gpus/. The market is competitive, with more than 100 GPU operators competing for customers. Providers offer various consumption models (on-demand, spot, contract/reserved) to match customer needs, and competition will only increase as this sector evolves. Optimizing and reducing power costs will become another differentiator for these operators.
GPU capacity will ultimately be traded on routers with the sophistication of an energy derivatives market. And in many ways, that's exactly what it is. For a GPU cluster to be competitive they must maneuver the router market, and the key advantage will be understanding the real time power costs. To know their real time power costs, their data centers need to understand the power costs, and the utility needs to provide the tools and products to make real time power prices available.
The strong market economics of power-aware GPU clusters
If GPU clusters can see their true, real-time power cost, they will start shedding volume when power costs are high, and undercutting their peers when power is cheap. The extreme power price periods are only for a few hours of the year, but the savings will give enormous advantage for the remaining hours. At 99% utilization, we have modeled power cost savings of 20% on the average power cost (using Australia's grid as an example). Cost savings can be booked as extra profit, because the expensive GPUs are still setting the market price higher.
This calculator shows the economics of a traditional GPU cluster using fixed pricing for power, versus a power-aware GPU cluster that modifies demand in peak periods.
There are profits for the taking
The calculator provides an illustration only, but we are seeing listed operators showing power and rent to be near the same cost as hardware depreciation, making this a reasonable assumptioniFY24: (73.4) Cost of Revenue / (77.3) Depreciation and Amortisation = 94.5% Nebius Annual Report 2024
FY24: (493.35) Cost of Revenue / (960.68) Technology and Infrastructure = 51.3%
Note: Coreweave's capacity is rapidly scaling, growing 8-fold over the year, so technology costs would lead revenues and lower this ratio, obscuring the economics
Coreweave Annual Report 2024. Again, we only have to look to Bitcoin mining for an indication of what the economics could look like as AI chips mature. Numbers from Bitcoin miner IRIS Energy suggest power costs could amount to 150% of hardware costsiFY24: (81,605) Electricity / (50,650) Depreciation = 161% IRIS Energy Annual Report 2024.
Operating a GPU cluster is a low-return activity, purely because it is so competitive. Players can't differentiate much on the purchasing of chips or leasing of facilities, so there is strong price competition. Even small improvements in power cost can drive economics. For example, if a cluster is making a 5% profit margin, and power accounts for 30% of its costs, then reducing the power bill by 20% will see profits more than double.
Nvidia is selling $100 billion USD worth of chips every year. By 2030, the footprint of GPU clusters will be enormous — imagine how much economic value could be created by managing their power consumption efficiently. The market is evolving rapidly, and power-aware operations will become a key competitive advantage in this increasingly saturated ecosystem.
Open source models will drive adoption
We expect open-source AI models to be the first to adopt power-aware operating models, because there competition to provide capacity for them. The price competition will eventually bleed into closed source models too, because the cost savings on open source could induce volumes away from closed. For example, if given a Claude 3.7 coding task, DeepSeek R1 may perform worse on a single query, but be competitive once at a cheaper price. Eventually closed source operators will get sick of only running when power is expensive, and need to make their own optimizations too.
Software programming is a key high-intensity AI use case
...we are not far from the world where, in 3-6 months, AI is writing 90% of the code.— Dario Amodei, Anthropic
Software engineering is one of the key industries being disrupted by AI — the productivity gains to be found are enormous and only escalating. Programming is rapidly becoming a high-intensity AI use case, with individual programmers spending thousands per year on agentic models that can complete entire coding tasks. At the current rate of improvement, AI coding agents will be capable of all programming tasks within the SWE benchmark (and that's no mean feat) by the end of 2025. Of course there are more difficult tasks that won't fall within this benchmark, but eventually we expect LLMs will be able to tackle them too.
As AI coding agents become more capable, they will be given larger and larger tasks. Today, agentic models are accomplishing tasks that take 30 seconds to two minutes. AI will eventually be given tasks to run for longer periods — potentially hours — coming back with complete, rigorously tested applicationsi"I think the direction that we, want our models to go in is being able to accomplish more and more complex tasks with as much autonomy as possible", Cat Wu, 6:29
https://youtu.be/zDmW5hJPsvQ?si=DaUb7su73MGi8hJX. Software engineers will no longer code, they will supervise.
The software development lifecycle will shift from two-week sprints to single day marathons. More of the human element will be scoping, specifications, business analysis. More of the software development will be automated and long running AI. The pattern will be the development team working in the morning, planning the prompts. The prompts will run at lunchtime - when power is cheap. Then there will be analysis and review in the afternoon, before sign off and deployment.
Agentic coding makes heavy use of routers, and is quickly dominating AI usageiAnthropic showed 37% of all queries are related to computer and mathematical:
Anthropic Economic Index
Within software development, Claude Code makes up more than Claude.ai:
Anthropic Economic Index: AI's Impact on Software Development. Competition is fierce, therefore the most cost effective platform will likely win most of the volume. There are numerous AI coding tools such as Cursor, Windsurf, RooCode, etc., all of which are using routing to some degree already. GPU clusters will fight to win the revenue that will go through routers, and they will use dynamic pricing with power awareness to take share. The duck curve will give periods where a power-aware cluster can significantly undercut peers, and periods where it does not make sense to deliver energy.
How to practically make GPUs aware of power costs
For all of this to come to fruition, GPU clusters need to integrate the duck-curve power price signals into their operating models, through an orchestration platform. GPU clusters are typically powered by SLURM or Kubernetes, which would need a module to integrate and provide signals based on business rules. If the GPU is tending capacity out to a router, they too will need a dynamic pricing system that takes the power prices into account.
Therefore, the data center that hosts the GPU cluster has to be capable of metering both usage and time-of-use, and reconciling against loss-of-effectiveness factors, such as missing data, for example. This is beyond the scope of most Data Center Infrastructure Management platforms or Energy Management Systems. There needs to be a specialized system to handle these aspects of power accounting, plus, ideally, a direct connection to the GPU cluster to ensure it all aligns.
Last but not least, the energy utility must have pricing and billing systems capable of providing these products to the data center, as well as hedging and sending signals down the supply chain. Most billing systems are barely capable of smart metering, let alone the kind of orchestration required to enable GPU clusters in this manner.
For this reason, currently, the only power-aware computing clusters run within their own facilities. Colocation facilities are left in the dark, and if they don't evolve they're at risk of being left behind by the AI boom.
The practical solution requires a unified platform to join the utility, the data center and the GPU cluster in harmony. That is what we're building at FlexSys.
AI can help us meet our emissions goals
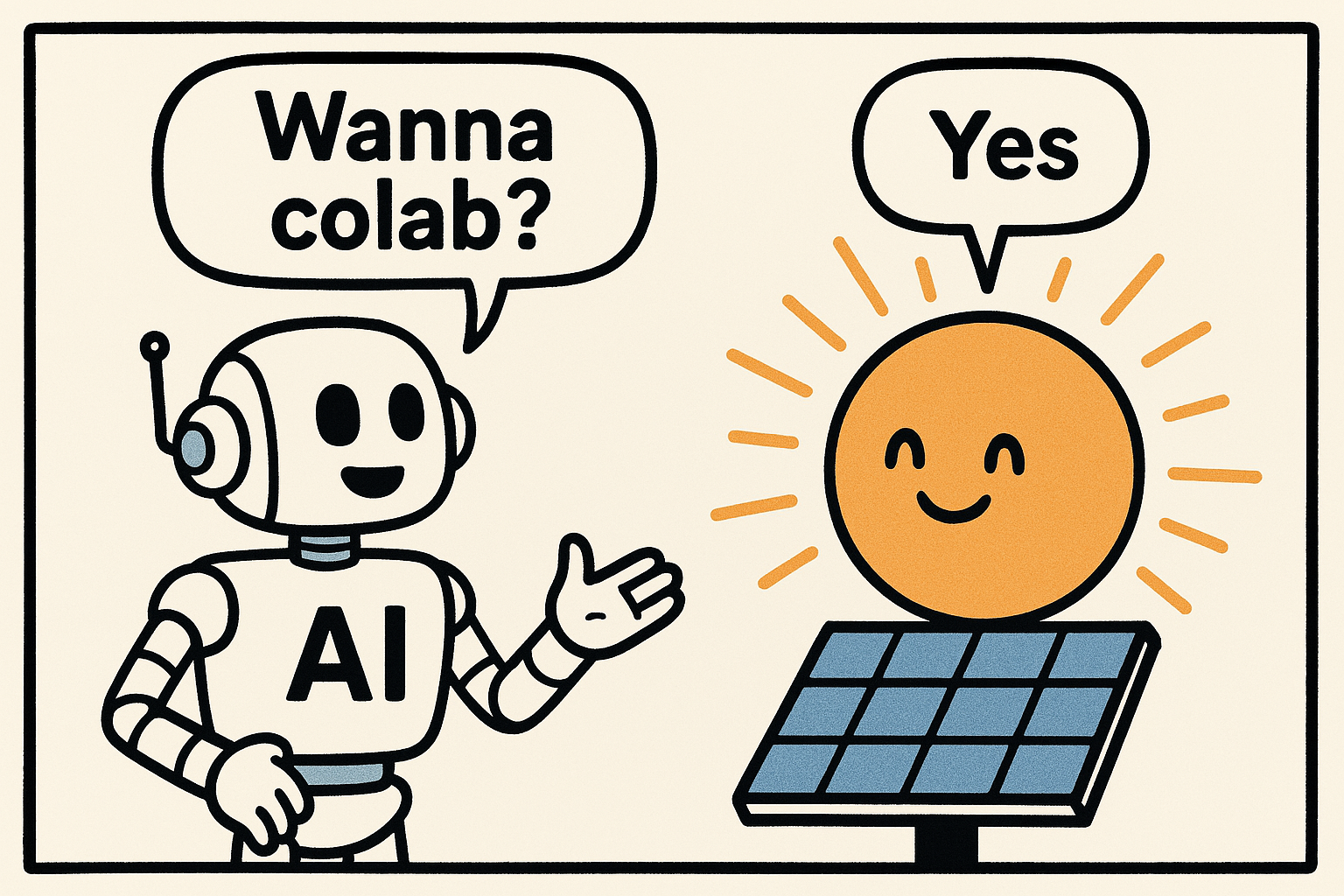
If 300GW of data center capacity could be completely run on renewables, that enables 1-2TW of additional renewable capacityiAssuming a capacity factor of ~15% to ~30% for renewables . This extra 1-2TW of renewable capacity would be 1-2 trillion USD worth of investment.iAssuming $1 USD per Watt . Assuming the data centers are already 20% renewable, the incremental improvement in emissions reduction would cut total CO2 emissions globally by 15%iEmissions reduction if displacing coal @ 3.5Mt CO2 per GW against 2TW of new wind. 7Gt out of 37.4Gt globally, 18.71%
Assuming 20% renewable generation currently, 18.71% * 80% = 14.97%
https://www.iea.org/data-and-statistics/charts/annual-direct-co2-emissions-avoided-per-1-gw-of-installed-capacity-by-technology-and-displaced-fuel. This impact could be multiplied further, as power-aware AI not only directly uses clean energy but also creates the grid flexibility needed to deploy significantly more renewable generation that benefits all electricity consumers.
It may seem an unlikely solution, but creating systems where large power consumers like AI data centers can directly respond to power price signals, and shift their workloads to times when electricity is abundant and cheap, actively balances the grid. We can make better use of renewable energy and reduce demand on the grid in peak times too, therefore reducing the need for fossil fuel.In the context of the energy transition, climate-aware computing is not a nice-to-have. It's a necessity.
Summary: Power-aware pricing is the future for powering AI
The Power Crisis Demands Smarter Consumption
The power crisis will force data centers and GPU clusters to evolve their power usage patterns. There is no other way to secure enough power for our AI ambitions. As grid capacity limits are reached globally and traditional baseload power sources diminish, AI providers who respond dynamically to power availability will gain competitive advantage while others face operational constraints.
Economics Heavily Favor Power-Aware Operations
High-intensity use cases like AI programming will realize significant benefits from power awareness. Even small improvements in power cost can dramatically impact profitability – a 20% reduction in power costs can more than double profits for operators running on thin margins. As GPUs depreciate faster with each product cycle, power becomes the dominant cost factor, creating a compelling economic case for facilities that can shift workloads to periods of renewable surplus.
A New Technical Platform Is Required
A unified system must link power utilities, data centers, and GPU clusters to enable responsive AI demand. Current infrastructure management systems aren't designed for this level of orchestration. The solution requires real-time pricing signals, sophisticated metering capabilities, and dynamic resource allocation – creating both challenges for legacy operations and opportunities for forward-thinking infrastructure providers.
Climate Impact Creates Additional Value
Once AI evolves its power demand patterns, the energy transition can accelerate significantly. By enabling 1-2 TW of additional renewable capacity through flexible consumption, power-aware AI could help reduce global CO2 emissions by up to 15%. This creates alignment between business interests and environmental goals, opening access to ESG-focused capital and regulatory advantages.
First Movers Will Capture Disproportionate Benefits
Early adopters of power-aware AI infrastructure will establish lasting competitive advantages. As the router-based LLM economy matures, providers offering lower costs during renewable-rich periods will capture greater market share. This creates a virtuous cycle where increased utilization further improves economics, potentially reshaping the competitive landscape between closed and open-source AI providers.
The world is changing, and we need energy infrastructure to change with it. A new platform is required to empower GPUs and data centers to avoid peak power usage, and for energy retailers to pass on flexible pricing capabilities.
If we can close that gap — if AI companies can execute high energy-demand activities at times of equally high supply; if programmers can schedule code to run over lunch — then we open up a world of possibilities. Smart use of power will be the defining factor of success in the AI sector.
Through innovation, evolution, and tailoring demand to supply, AI can genuinely play a major role in turning the power crisis around. What initially appeared as a problem—the energy-intensive nature of AI—may ultimately become part of the solution to our broader energy and climate challenges.
This is necessary, globally, if we're going to meet our climate goals — and for economies, for society and for humanity as a whole.
Discover how our intelligent energy solutions can optimize your AI infrastructure. Contact us at corporate@flipped.energy.